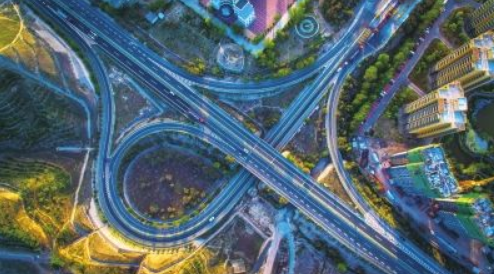
The impact of transportation infrastructure on the economy development based on the Yangtze River Delta region
经济paper代写 This paper's main research content is the relationship between transportation infrastructure and regional growth in the
Abstract 经济paper代写
The investment in the transportation infrastructure plays a vital role in the development of economy. In this paper, we use the data from 2007 to 2017 in three Chinese provinces, Zhejiang, Jiangsu and Shanghai. We apply the spatial econometric model to analyze the spatial correlation between the growth of economy and the development of transportation infrastructure. Our findings show that there is a positive spatial correlation between the transportation and economy. Also, we find that the traditional factors, such as fixed assets investment, human capital are also affected by the transportation. The impact of transportation infrastructure on the economy can provide us with evidence of potential policy for local government. The local government should not only develop its own infrastructure but also need to pay attention to the transportation of its neighbors.
Key words: China; transportation infrastructure; spatial autocorrelation; economic growth
Introduction 经济paper代写
As a prerequisite for regional development, transportation infrastructure is the foundation of regional economic growth, and it plays a significant role in achieving coordinated development between regions. A perfect transportation network has improved the regional industrial layout and development model, reduced the cost of transit and trade between regions, and significantly promoted production factors between different regions. Therefore, the relationship between the construction of transportation infrastructure and the macro-regional economy development is inseparable.
A large part of China's fiscal expenditure is used for investment and construction of transportation infrastructure. From 2007 to 2017, the investment expenditure on transportation infrastructure in the Yangtze River Delta has increased from 14 billion to 123.1 billion annually, rising sharply year by year. Correspondingly, the transportation industry's total infrastructure fixed assets in the Yangtze River Delta region rose from 42.4 billion to 681.8 billion, and the growth trend was rapid.
The construction of transportation infrastructure plays an irreplaceable and vital role in promoting the Yangtze River Delta's integrated development.
At present, the transportation planning and construction of cities are based more on the city's considerations. However, the reality is that the construction of transportation facilities in this region will affect its own economic growth and have specific spillover effects on surrounding cities. This part is often ignored. In practice, developed regions have formed a relatively complete transportation network system, gathering high-quality talents, production factors, and innovative technologies to become the core of economic growth.
The backward regions will lose their high-quality production factors, which will further widen the gap between regions. Therefore, in the context of regional integration in the Yangtze River Delta, the investment in transportation infrastructure must be based on the region's coordinated development, break the boundaries and barriers between each other, and achieve inter-regional interconnection and joint development. This paper studies the spillover effects of transportation infrastructure between regions, which is conducive to enhancing the effectiveness of existing planning policies and promoting the coordinated development of regional integration in the Yangtze River Delta.
China has long faced different inclination of resource allocation, uneven distribution, and a massive regional development gap. 经济paper代写
The transportation network system is characterized by dense east and west sparseness and significant differences in density. The Yangtze River Delta region, as the most representative urban agglomeration in China's modernization drive, is a critical intersection between the One Belt One Road and the Yangtze River Economic Belt, and it continues to promote the effectiveness of trade and development relevance in the regions along the One Belt, One Road.
Therefore, studying the spatial effects of transportation infrastructure construction in the Yangtze River Delta region on the regional economy is not only a specific study of the interaction between transportation infrastructure and economic growth in the Yangtze River Delta region but also hopes that The role experience is used to guide the development of the surrounding underdeveloped areas, thus showing the critical role of transportation infrastructure construction in my country's regional economic growth.
Finally, because China's regional delineation of Anhui Province was not clear enough before 2014, although it has now formally joined the Yangtze River Delta urban agglomeration, to ensure the continuity, completeness, accuracy, and availability of data, this article is When selecting the data, the data of Anhui Province is presented.
Literature
2.1 The impact of transportation infrastructure investment on economic growth
The construction of transportation infrastructure breaks the barriers between regions. It promotes the flow and return of production factors such as labor, capital, and technology among different regions, conducive to promoting technological progress and economic growth between regions. Adam Smith once pointed out that the improvement of transportation infrastructure construction is conducive to promoting the market's continuous development and affirmed the critical role of transportation conditions in economic development.
Friedrich (1841) also emphasized that its economic production capacity is directly related to its transportation capacity. The more developed the transportation, the higher the production level. At the same time, it shows that the government plays an essential role in constructing transportation infrastructure. Appropriate intervention and reasonable planning are critical.
Based on this, more and more scholars are devoted to analyzing the relationship between the two, combining qualitative and quantitative, and endless economic theories have emerged. Aschauer(1991) constructed a spatial measurement model and conducted empirical research concerning the United States' economic data from 1949 to 1985. It concluded that investment in the transportation industry is positively promoting economic growth, with an impact of 0.93%.
Pradhan (2010) conducted an empirical analysis on the time series data of all cities in India from 1970 to 2007 and found through the Granger causality test method that transportation infrastructure and regional economic growth are a long-term one-way Granger causal connection. Mahbubu Hakim and Rico Merkert (2016), based on the air transportation data of 8 countries in South Asia from 1973 to 2014, through co-integration analysis and impulse response analysis, found that air transportation has a significant role in promoting economic growth, but its impact needs to be compared. It takes a long time to show up, and there is a one-way causal relationship between the two.
2.2 Research on the Spatial Effect of Transportation Infrastructure Investment 经济paper代写
Hohz Eakin and Schwartz (1995) added spatial elements to the traditional analysis, constructed the most initialized spatial adjacent weight matrix, and tested whether infrastructure such as transportation has spillover effects on economic growth. Econometric analysis based on the highway data of 48 states in the United States in 18 years and finally found that the impact effect is not significant. Boarnet (1999) built a spatial econometric model and conducted an empirical analysis of all California counties' panel data in the United States from 1969 to 1988 and found that highways in the transportation infrastructure have a negative spillover effect on economic growth.
However, studies by scholars such as Periraand Sagales (2003) and Cohen (2007) show that the construction of transportation infrastructure has a significant spillover effect on the surrounding areas' economic growth. It is a positive spillover. Ozbay (2007) and others selected 10-year traffic and economic data in New York and New Jersey. They found through spatial measurement empirical evidence that there is a spatial spillover effect in transportation facilities' investment. As the distance increases, the intensity of the spillover effect gradually weakens. However, Due to the long transportation construction period, there is a certain lag in its effect to take effect.
The Model
3.1 Spatial autocorrelation
Spatial autocorrelation is a particular form of spatial dependence, which can measure whether the variables in the regions close to each other are similar. If the change of a particular variable in a particular area has the same or similar trend with other neighboring areas, it is called positive spatial autocorrelation. On the contrary, it is called negative spatial autocorrelation. If the distribution is random, there is no spatial autocorrelation. This section will use exploratory spatial statistical methods to test whether there is such a correlation in the Yangtze River Delta. The most popular method for measuring spatial autocorrelation is Moran's Index.
The mathematical expression of Moran's Index I am,
where is the sample variance, n is the number of the areas, w is the weighted spatial matrix. Denotes the total sum of all the spatial weights. If we standardize the spatial weight matrix, then The Moran's Index transforms as,
Moran's Index's value is between -1 and 1. A value is greater than 0 means that there is a positive spatial correlation among areas, 0 means that the spatial distribution is random and smaller than 0 means a negative spatial autocorrelation. As Moran's Index follows a distribution with an expectation of E(I) and Var(I) variance, we can construct a standardized Z test to test the significance of spatial autocorrelation between areas.
3.2 Partial Spatial Autocorrelation
Partial spatial correlation is also used to analyze the existence of similarities and differences in some areas. The usual measurement is Local Moran's I. It is given by,
Where n denotes the total number of areas, w is the weighted spatial matrix, and denotes the observed value in area j and area i, respectively. A positive number means a positive local autocorrelation, and a negative one shows a negative local autocorrelation.
3.3 Spatial autocorrelation matrix
We usually define a binary symmetrical spatial weight matrix to represent the proximity relationship between n regions in research. Its common expression is as follows,
Where n denotes the number of regions, Represents the distance between region i and region j. The main diagonal element is zero; that is, the distance between the same area is zero. The distance setting in different spatial weight matrices has many forms, and different variable distances can be incorporated according to specific research problems, such as economics, economic geography, etc., to give different meanings to distances, making the distance more practical and realistic significance. Based on previous literature, we have two types of the spatial weight matrix.
3.3.1 Binary critical space weight matrix, W1
If area i and area j are adjacent, the weight is 1; otherwise, it is 0 if they are not adjacent.
3.3.2 Inverse distance space weight matrix, W2
The geographic distance between regions can represent the relationship between regions, and the relationship between the regions and the increase in the distance between regions have a reversed relationship. Therefore, we can use the reciprocal power of the geographic distance between regions as the weight element to construct a weight matrix. If we use d representing the geographical distance between areas, the matrix can be expressed by,
Spatial econometric model 经济paper代写
- Spatial Lag Model
Y is the dependent variable, X is the vector of independent variables, W is the spatial weight matrix, WY is the dependent variable's spatial lag term. is the spatial autocorrelation coefficient, is the coefficient of the explanatory variables, and is the normally distributed error term.
Spatial Error Model
Y is the dependent variable, X is the vector of independent variables, W is the spatial weight matrix. is the spatial error autocorrelation coefficient, is the coefficient of the explanatory variables, and is the normally distributed error term.
Spatial Durbin Model
Durbin Model is a modification of the spatial lag model. It includes the spatial lag term of the explanatory variable. When gets different values, it can be transformed into a spatial error model or the spatial lag model.
Data Description
The data set spans the period 2007 to 2017. I draw from the data set the following variables:
Dependent variable: GDP per capita, an indicator of regional development level.
Independent variables:
- urb, an indicator of urban population ratio.
- Fai, an indicator of fixed assets investment.
- Res, an indicator of technology expenditure.
- Emp, an indicator of labor input level.
- Edu, an indicator of the human capital level.
- Gov, an indicator of government intervention.
- Dummies: year dummy
Table 1 reports the summary statistics for the key variables.
Table1: Summary Statistics | ||||||
Obs | Mean | Sd | Min | Median | Max | |
Gdp | 275.0 | 74016.85 | 23584.80 | 34198.00 | 73002.00 | 127000.00 |
Urb | 275.0 | 71.11 | 13.20 | 53.20 | 65.80 | 89.60 |
Fai | 275.0 | 17698.67 | 12987.42 | 4458.61 | 14077.25 | 49370.85 |
Res | 275.0 | 1940000.00 | 883000.00 | 498000.00 | 2150000.00 | 3400000.00 |
Emp | 275.0 | 0.58 | 0.17 | 0.25 | 0.60 | 0.77 |
Edu | 275.0 | 10.94 | 1.11 | 9.40 | 11.17 | 13.06 |
Gov | 275.0 | 5234.62 | 2425.57 | 1806.79 | 4730.47 | 10621.03 |
Plot 1 shows the tendency for the main independent variables and time and the dependent variable GDP per capita.
Plot 1: The correlation between variables
Also, I'd like to have a look at the comparison between the three provinces. Plot 2 shows the change of GDP per capita in three provinces from 2007 to 2017.
Plot 2: The tendency of GDP per capita in three provinces
Plot 3 shows the other variables in three countries.
Plot 3: The tendency of main independent variables
Results 经济paper代写
5.1 LM test
Before I run the regression, I first do the LM test. Based on the LM test result, I will choose the best one from our three models. Thus, I used four LM tests, including LM-lag, LM-error, robust LM-lag, and robust LM-error. The results are as follows,
Table 2: LM Test Result | ||
LM statistics value | P-value | |
LM-lag | 37.468 | 0.000 |
LM-error | 54.162 | 0.000 |
Robust LM-lag | 39.550 | 0.000 |
Robust LM-error | 65.240 | 0.000 |
The LM statistics are 37.468, 54.162, 39.550, and 65.240 for LM-lag, LM-error, robust LM-lag, and robust LM-error test under the adjacent matrix, respectively. The p values are all more minor than 0.0001. It means that they are significant at 1% level. The data have both the lag term and the error structure. Hence, I will apply both the spatial error model and the spatial lag model.
5.2 Hausman test
Since my data is a panel data set, I need to test for endogeneity. I use the most common method to test for it, the Hausman test. The result is as follows,
Table 3: Hausman Test Result | ||
Statistics value | P-value | |
Hausman | 34.134 | 0.000 |
The result shows that the test is significant at 99% level. There is endogeneity in our data. To eliminate this effect, I choose to use the fixed-effect model. As our model also includes the spatial regression factors. I will do a spatial fixed-effect regression.
5.3 Global autocorrelation of the growth of the Yangtze River Delta
According to the theory of spatial laws in geography, the surrounding areas' economic development has been determined to be closely related to the central area rather than exist in isolation. According to the accessibility mentioned above, the spatial statistical analysis method, the three provinces in the Yangtze River Delta Economic Zone are first analyzed for spatial correlation. The global spatial autocorrelation test is first performed. Calculate the Moran index of per capita GDP in the Yangtze River Delta region based on a geographically critical binary weight matrix,
Table 4: Moran Index for GDP per capita | ||
Year | Moran's Index | P-value |
2007 | 0.499 | 0.001 |
2008 | 0.498 | 0.003 |
2009 | 0.421 | 0.001 |
2010 | 0.465 | 0.001 |
2011 | 0.495 | 0.001 |
2012 | 0.498 | 0.002 |
2013 | 0.463 | 0.002 |
2014 | 0.498 | 0.002 |
2015 | 0.496 | 0.001 |
2016 | 0.481 | 0.001 |
2017 | 0.498 | 0.001 |
On the whole, in the study period from 2007 to 2017, the Moran Index in the Yangtze River Delta Economic Region is positive, which shows a significant spatial correlation, and the Moran Index remains stable in the 0.4 to 0.5 interval. Furthermore, the Yangtze River Delta's economic region is not randomly distributed in space but presents an apparent spatial aggregation state, and there is a spatial effect.
5.4 Main regression result 经济paper代写
Table 5: Spatial panel regression results | ||||
OLS | SAR | SEM | SDM | |
Fai | -0.698*** (-21.703) | 0.393*** (12.220) | 0.158*** (7.751) | 0.137*** (8.692) |
Emp | 0.170*** (7.846) | 0.226*** (10.431) | -0.076*** (-5.826) | -0.042*** (-3.351) |
Edu | 0.178*** (8.206) | 0.118*** (5.834) | 0.090*** (5.997) | 0.086*** (6.920) |
Urb | 0.386*** (9.580) | 0.238*** (5.900) | 0.065*** (3.951) | 0.215*** (5.532) |
Res | -0.000 (-0.012) | 0.180*** (4.301) | -0.036*** (-2.621) | 0.031 (1.139) |
Gov | 0.5591*** (14.764) | 0.253*** (6.681) | 0.025 (1.526) | -0.042 (-1.473) |
rho | - | 0.6453*** (17.041) | 0.7416*** (45.267) | 0.8533*** (29.92) |
R-sq | 0.9916 | 0.9766 | 0.9777 | 0.9810 |
*all regressions contain a complete list of year dummies
From the estimated results in the table, it can be seen that the regression result coefficients of the three spatial measurement models under the adjacency matrix are significant, indicating that the economic growth level of a single city will be affected by the economic activities of other cities. From the simulation effect, the SDM model is better than the SAR and SEM models, which further verifies the conclusion of the LR test.
The economic output of transportation infrastructure construction is positive. It is significant at the 95% confidence level, indicating that the transportation infrastructure and regional economic growth are positively correlated. The more complete the transportation infrastructure, the correspondingly faster promote economic growth. The further comparison found that the coefficients of fixed assets, human capital, scientific research output, and other variables are all positive and significant under the 95% confidence interval, so I conclude that these traditional production factors still have a significant role in promoting economic development. Under the new economic normal, the Yangtze River Delta region's economic development still needs to pay attention to and rationally carry out capital investment and personnel training to stabilize economic growth's fundamental driving force. However, the effect of research investment is not significant at a 95% significant level. It means that the marginal effect of research input is not significant on the growth of the economy. This issue can be explained that the R&D investment in these regions is already very high, so that the marginal return tends to be lower.
Conclusion 经济paper代写
This paper's main research content is the relationship between transportation infrastructure and regional growth in the Yangtze River Delta and its spatial spillover effects. From a spatial perspective, based on Jiangsu, Zhejiang and Shanghai's panel data from 2007-2017, a spatial econometric model is constructed. The empirical analysis results are as follows:
1.There is significant spatial correlation and heterogeneity in economic growth in the Yangtze River Delta. However, there is a particular gap in regional growth, and the layout of transportation infrastructure is unreasonable and uneven, and there are differences.
2.There is a significant positive correlation between transportation infrastructure construction in the Yangtze River Delta and economic growth. The output elasticity of transportation infrastructure to the economy is about 0.02. Compared with the traditional OLS model, the direct effect of transportation infrastructure is smaller than its estimate. The coefficient, that is, without considering the spatial impact, the traditional OLS overestimates the impact of local transportation infrastructure on the regional economy.
3.The transportation infrastructure has a spillover effect on regional economic growth, and the spillover effect is significantly harmful. The local transportation infrastructure will produce a siphon phenomenon, causing the loss of production factors in surrounding cities. This has caused the gap between regions to continue to widen.
4.Traditional production factors, such as capital and labor, still play a significant role in promoting economic growth. However, the new economic growth factors, human capital, urbanization, and innovation degree are also increasingly important to the economy. It shows that the transportation network's improvement has dramatically promoted the circulation and return of multiple production factors in various regions, increased the links between regional economic activities, and expanded the influence of various factors that are conducive to economic growth, especially the new economy. The influence of growth factors cannot be ignored.
Based on our findings, we also propose several policy recommendations to boost the economy.
1.Space factors should be fully considered in the planning of transportation infrastructure in the Yangtze River Delta. There is significant spatial correlation and heterogeneity in the economic growth of the Yangtze River Delta region. Therefore, when planning the Yangtze River Delta region's transportation infrastructure construction, the government must fully consider spatial influence factors, attach importance to the links between economic activities in various regions, and promote overall unified planning. At the same time, we must also pay attention to the transportation planning of various regions to truly break the boundaries between various regions and realize interconnection and regional economic integration.
2.The Yangtze River Delta region still needs to increase investment in transportation infrastructure. Increase the importance of coordination in the three aspects of transportation infrastructure investment, planning, and construction, and improve the networked transportation process of roads, railways, waterways, and aviation.
3.Strengthen the cultivation of economic growth points in non-core regions of the Yangtze River Delta. To reduce the outflow of high-quality talents and resources and avoid the negative impact of negative spillovers from core areas, non-core areas need to find new economic growth points, strengthen talent training, urbanization construction, and innovative research and development, and continuously enhance the attractiveness of their cities. Simultaneously, in the process of economic agglomeration, each region must strengthen the differentiation and uniqueness of its development, form a comparative advantage, avoid blind and homogeneous competition, and narrow the gap in regional economic development.
Reference 经济paper代写
Boarnet, M. G. (1999). Road Infrastructure, Economic Productivity, and the Need for Highway Finance Reform. Public Works Management & Policy, 3(4), 289–303. https://doi.org/10.1177/1087724x9934002
David Alan Aschauer, 1991. "Infrastructure: America's Third Deficit," Challenge, Taylor & Francis Journals, vol. 34(2), pages 39-45, March.
Hakim, M. M., & Merkert, R. (2016). The causal relationship between air transport and economic growth: Empirical evidence from South Asia. Journal of Transport Geography, 56, 120–127. https://doi.org/10.1016/j.jtrangeo.2016.09.006
Holtz-Eakin, D., & Schwartz, A. E. (1995). Infrastructure in a structural model of economic growth. Regional Science and Urban Economics, 25(2), 131–151. https://doi.org/10.1016/0166-0462(94)02080-z
Ozbay, K., Ozmen-Ertekin, D., & Berechman, J. (2007). Contribution of transportation investments to county output. Transport Policy, 14(4), 317–329. https://doi.org/10.1016/j.tranpol.2007.03.004
Pereira, A. M., & Roca-Sagalés, O. (2003). Erratum to "Spillover effects of public capital formation: evidence from the Spanish regions." Journal of Urban Economics, 54(1), 197. https://doi.org/10.1016/s0094-1190(03)00064-0
Pradhan, S. (2010). Catalyzing Change through Innovation. Development Outreach, 12(1), 2–3. https://doi.org/10.1596/1020-797x_12_1_2
发表回复
要发表评论,您必须先登录。